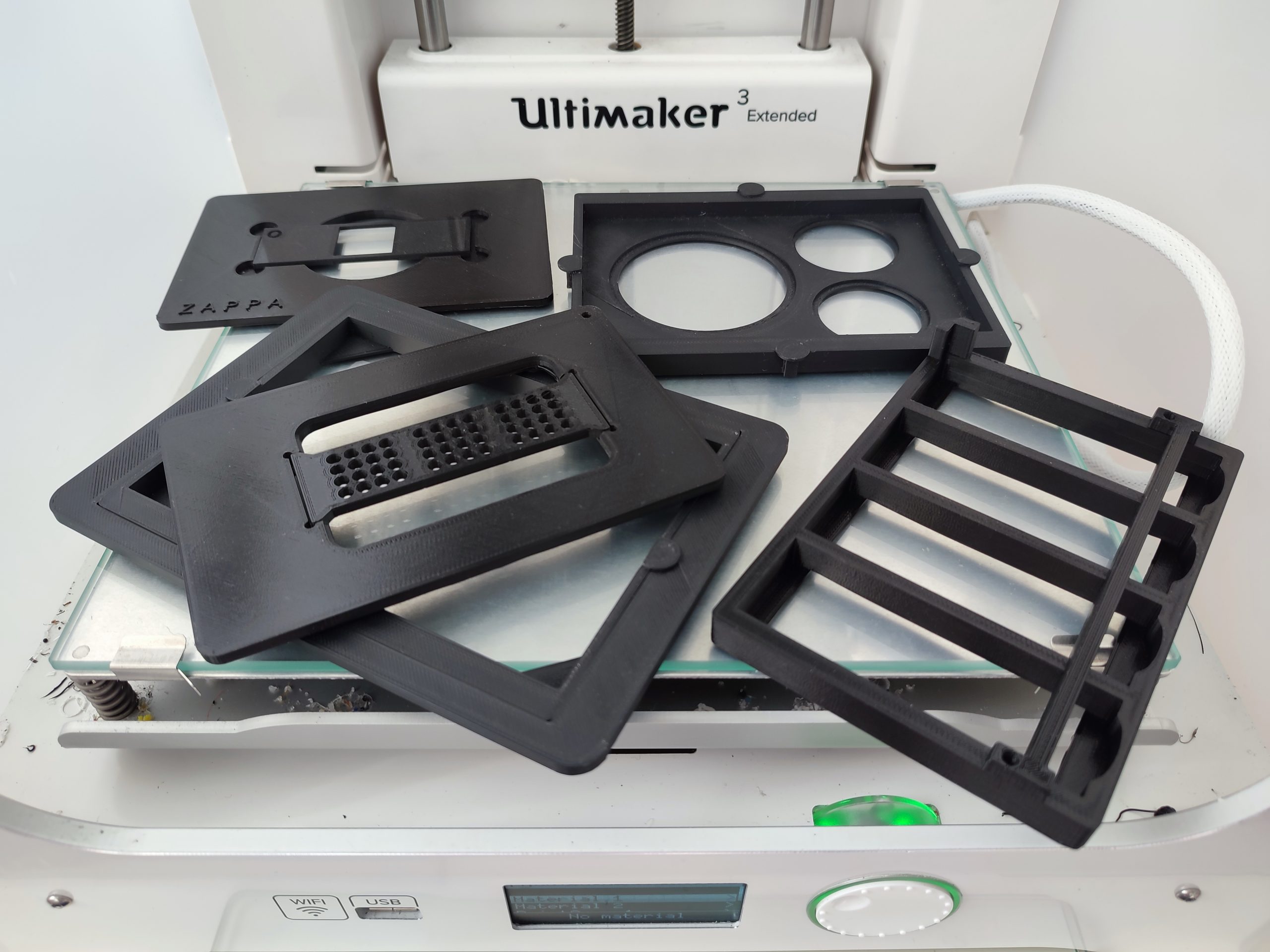
Above: A selection of stage plate inserts 3D printed by the Bioimaging Research Hub – links to resources in blog article below.
Hands up if your microscope is badly in need of upgrade or repair but your budget won’t stretch that far? Maybe a new focusing knob to replace the one that just broke off in your hand, or perhaps a new stage plate adapter, reflector cube or filter holder to increase your imaging options? Perhaps a C-mount or smartphone adaptor to give one of your old microscopes a new lease of life? Or even a sample holder or chamber for a bespoke imaging application? What the heck, let’s think big eh? How about a completely new modular microscope system with tile scanning capabilities?
Way too expensive, eh?… Well, imagine for a moment that you could just click a button (or a few buttons, at least) and make it so. If you haven’t yet realised, I’m talking about 3D printing in light microscopy and the life sciences – the subject of a very interesting paper that I recently came across – see below.
It’s safe to say that 3D printing is changing the way we do things in microscopy, now permitting low-cost upgrade, repair, or customisation of microscopes like never before. There are now a huge selection of 3D printable resources available through websites such as NIH3D, Thingiverse etc that can be used to modify your microscope system or to generate scientific apparatus or labware for upstream sample processing and preparation procedures.
So, to save you trawling through the 3D printing sites in order to identify the most useful designs to meet your histology and imaging needs we’ve done it for you and have curated a list of 3D printable resources which we hope you you’ll find useful (below).
AJH 25/05/2023
Further Reading
- Rosario, D. et al. (2021) The field guide to 3D printing in optical microscopy for life sciences. Advanced Biology. 6: 2100994.
—————————————————————-
3D printable resources for histology and light microscopy. Information collated by Dr Tony Hayes, Bioimaging Research Hub, School of Biosciences, Cardiff University, Wales, UK.
Sample processing
- A 3D printable plastic trough for serial semithin sectioning: https://www.thingiverse.com/thing:3427208
- Brain slide adapter 2mm: https://www.thingiverse.com/thing:5529501
- Handheld microtome: https://www.thingiverse.com/thing:2178524
- Histology plate 20 well v1: https://3d.nih.gov/entries/3DPX-011746
- Histology tray 10 well v1: https://3d.nih.gov/entries/3DPX-011747
- Histology tray 20 well v1: https://3d.nih.gov/entries/3DPX-011748
- Histolology plate 10 well v1: https://3d.nih.gov/entries/3DPX-011745
- Mouse brain matrix coronal 0.5mm: https://www.thingiverse.com/thing:4339995
- Mouse brain matrix coronal tool 1mm: https://www.thingiverse.com/thing:4339985
- Mouse brain matrix sagittal 0.5mm: https://www.thingiverse.com/thing:4339999
- Mouse brain matrix sagittal 1mm: https://www.thingiverse.com/thing:4350604
- Mouse brain matrix: https://www.thingiverse.com/thing:3077272
- Pathology box for grossing small samples: https://www.thingiverse.com/thing:5800585
- Tissue array mould for processing punch biopsies: https://www.thingiverse.com/thing:5529420
- Tissue fixation anchor: https://www.thingiverse.com/thing:2910937
Sample staining
- 12 well plate trans-well tray for staining: https://www.thingiverse.com/thing:1761733
- 22x22mm glass coverslip holder: https://www.thingiverse.com/thing:29422
- Auto-stainer for biological specimens 3D printed USB powered: https://www.thingiverse.com/thing:282556
- Immunohistochemistry staining chamber for medical research (2 slide holder): https://www.thingiverse.com/thing:4314593
- Immunohistochemistry staining chamber for medical research (8 slide holder): https://www.thingiverse.com/thing:4314587
- Immunostaining box – 16 slides: https://www.thingiverse.com/thing:3080634
- Immunostaining box: https://3d.nih.gov/entries/3DPX-012172
- Lab slide dryer rack: https://www.thingiverse.com/thing:4344105
- Segmented interlocking well spacer for UARM-Pro experimental pathology stainer/deparaffinization platform: https://www.thingiverse.com/thing:2318519
- Slide staining rack: https://www.thingiverse.com/thing:44824
Sample storage and archiving
- Coverslip rack: https://www.thingiverse.com/thing:2091269
- Microscope cover slip holder: https://www.thingiverse.com/thing:4865521
- Microscope double coverslip holder slide: https://www.thingiverse.com/thing:2791356
- Microscope slide box – 20 slides: https://www.thingiverse.com/thing:3610374
- Microscope slide holder – 45 slides: https://www.thingiverse.com/thing:2720290
- Microscope slide holder (10 slides flat, with magnetic latch): https://www.thingiverse.com/thing:4314596
- Microscope slide holder: https://www.thingiverse.com/thing:3997537
- Microscope slide holder: https://www.thingiverse.com/thing:4823548
- Microscope slide storage styled on Sir Sherrington’s histology box: https://www.thingiverse.com/thing:2462523
- Microscopy slide box (25 slides): https://www.thingiverse.com/thing:3297002
- Microscopy slide carrier: https://www.thingiverse.com/thing:4642421
- Parametric microscope slide holder: https://3d.nih.gov/entries/3DPX-000746
- Transport box for 10 microscope slides: https://www.thingiverse.com/thing:5023250
- SEM specimen holder rack: https://www.thingiverse.com/thing:2765169
Sample presentation
- 5o angle cuvette: https://3d.nih.gov/entries/3DPX-014299
- 45o angle cuvette: https://3d.nih.gov/entries/3DPX-014300
- 5 o angle cuvette: https://3d.nih.gov/entries/3DPX-014328
- Flat solid sample cuvette: https://3d.nih.gov/entries/3DPX-014330
- Flat solid square sample cuvette: https://3d.nih.gov/entries/3DPX-014331
- Flat solid triangle sample: https://3d.nih.gov/entries/3DPX-014332
- Histology slide holder for polishing thin sections: https://www.thingiverse.com/thing:4751697
- Holder for baby zebrafish: https://www.thingiverse.com/thing:2803021
- Insect specimen holder: https://www.thingiverse.com/thing:5324399
- Microchamber slide for confining motile cells during imaging: https://3d.nih.gov/entries/3DPX-017460
- Microscope slide (25x75mm) with inset (22x22mm): https://3d.nih.gov/entries/3DPX-009765
- Microscope slide (25x75mm) with inset (22x40mm): https://3d.nih.gov/entries/3DPX-015994
- Microscope slide sample chambers and liquid rings: https://www.thingiverse.com/thing:3379473
- Microscopy chamber for Arabidopsis: https://www.thingiverse.com/thing:3710588
- PICS platform for wholemount imaging of live tissue: https://3d.nih.gov/entries/3DPX-009586
- SCORE imaging FEP tube holder: https://3d.nih.gov/entries/3DPX-000123
- TRIO imaging support platform for mice: https://3d.nih.gov/entries/3DPX-010720
- Visible root pot: https://www.thingiverse.com/thing:4738692
- Wet chamber/reaction room for microscopy applications: https://www.thingiverse.com/thing:2945057
- Zebrafish intubation chamber: https://3d.nih.gov/entries/3DPX-016689
Microscope: Complete builds
- A fully printable microscope: https://3d.nih.gov/entries/3DPX-000304
- Microscope: https://www.thingiverse.com/thing:4366390
- OpenFlexure microscope: https://openflexure.org/; refs: https://openflexure.org/about/media-publications
- Optical breadboard and toolset: https://www.thingiverse.com/thing:4637367
- PUMA microscope: https://github.com/TadPath/PUMA; ref: https://onlinelibrary.wiley.com/doi/10.1111/jmi.13043
- Raspberry Pi scope: https://3d.nih.gov/entries/3DPX-000609
- UC2 open and modular optical toolbox: https://github.com/openUC2/UC2-GIT; ref: https://www.nature.com/articles/s41467-020-19447-9
Microscope: Maintenance
- Binocular eyepiece caps: https://www.thingiverse.com/thing:3421681
- Eyeglass wipe holder: https://www.thingiverse.com/thing:4639749
- Eyepiece case for microscope eyepieces: https://www.thingiverse.com/thing:4445491
- Microscope objective case holder: https://www.thingiverse.com/thing:5167362
- Optics cleaning station: https://www.thingiverse.com/thing:2791398
- Zeiss lens cleaning wipe dispenser (needs spring): https://www.thingiverse.com/thing:4765397
- Zeiss lens wipe holder: https://www.thingiverse.com/thing:5236982
Microscope: Phone adapters (a selection)
- AmScope stereo microscope phone adapter: https://3d.nih.gov/entries/3DPX-012453
- Cell-phone rest for National 162 microscope: https://3d.nih.gov/entries/3DPX-012705
- iPad microscope adapter: https://www.thingiverse.com/thing:1622357
- iPhone 4 microscope holder: https://www.thingiverse.com/thing:718258
- iPhone 5 microscope adapter: https://www.thingiverse.com/thing:32312
- iPhone 5S mount for Zeiss Primo Star microscope: https://www.thingiverse.com/thing:4717495
- iPhone 6 microscope adapter: https://www.thingiverse.com/thing:679991
- iphone microscope adapter: https://3d.nih.gov/entries/3DPX-000491
- iphone4 microscope adapter 31mm diameter: https://www.thingiverse.com/thing:2863029
- Microscope phone mount: https://www.thingiverse.com/thing:5254019
- Microscope smartphone adapter customisable: https://www.thingiverse.com/thing:4145621
- Mobilephone microscope clip: https://3d.nih.gov/entries/3DPX-009111
- Phone stand for slide imaging: https://3d.nih.gov/entries/3DPX-012992
- Smartphone microscope mount (universal): https://www.thingiverse.com/thing:3474717
- Universal camera phone/microscope adapter: https://www.thingiverse.com/thing:78071
- Universal microscope phone adapter: https://www.thingiverse.com/thing:59344
- Universal phone microscope adapter: https://www.thingiverse.com/thing:431168
Microscope: Stands (a selection)
- C-mount adjustable microscope stand: https://www.thingiverse.com/thing:2546712
- C-mount adjustable microscope stand: https://www.thingiverse.com/thing:2611461
- Fully printable digital microscope stand: https://www.thingiverse.com/thing:2398466
- Microscope stand (bigger): https://www.thingiverse.com/thing:4845984
- Microscope stand: https://www.thingiverse.com/thing:4796754
- USB microscope stand: https://www.thingiverse.com/thing:4609785
- USB microscope stand: https://www.thingiverse.com/thing:5122033
Microscope: Components (generic)
- 10cm culture dish adapter plate: https://3d.nih.gov/entries/3DPX-010083
- 30mm adapter for 23mm ultra-wide microscope eyepiece: https://www.thingiverse.com/thing:4614915
- 32mm darkfield filter stop for microscopes: https://www.thingiverse.com/thing:3055364
- 32mm oblique filter stop for microscopes: https://www.thingiverse.com/thing:3055389
- 4-slide adapter for multi-well plate microscope holder: https://3d.nih.gov/entries/3DPX-012891
- A printable microscope focus-lock upgrade: https://www.thingiverse.com/thing:85698
- Camera microscope mount: https://www.thingiverse.com/thing:723227
- Coverslip chamber to slide adapter: https://www.thingiverse.com/thing:798161
- Darkfield condenser high NA: https://www.thingiverse.com/thing:5241935
- Darkfield microscopy filters: https://www.thingiverse.com/thing:5155864
- Darkfield/phase contrast/oblique illumination microscope set: https://www.thingiverse.com/thing:3067166
- Double dish adapter for microscopy: https://www.thingiverse.com/thing:3777647
- FDM-printable microscope objective RMS thread: https://www.thingiverse.com/thing:5400281
- Filter adapter for a digital microscope camera: https://3d.nih.gov/entries/3DPX-014498
- Fluorescence filter cubes (various manufacturers): https://www.thingiverse.com/thing:3639658
- Hoffman modulation contrast (HMC) DIY: https://www.thingiverse.com/thing:5881647
- Light diffuser for photomicrography (extreme macro) for vertical stand on petri dish large 40mm max: https://www.thingiverse.com/thing:4941862
- Light tight enclosure for microscope sample v.1: https://www.thingiverse.com/thing:1221320
- Microscope 3D print holders: https://3d.nih.gov/entries/3DPX-016965
- Microscope camera port adapter DM-SM1A50: https://3d.nih.gov/entries/3DPX-010946
- Microscope dish adapter: https://3d.nih.gov/entries/3DPX-000117
- Microscope eyepiece protection cap: https://www.thingiverse.com/thing:4828621
- Microscope eyepiece to camera adapter with cap: https://www.thingiverse.com/thing:4234443
- Microscope eyepiece to camera adapter with cap: https://www.thingiverse.com/thing:4234443
- Microscope RMS parfocal extender & M25: https://www.thingiverse.com/thing:4567179
- Microscope slide cooler: https://www.thingiverse.com/thing:2796001
- Microscope slide well plate adapter for live cell imaging: https://www.thingiverse.com/thing:5398732
- Microscope stage insert: https://www.thingiverse.com/thing:2754751
- Parametrised microscope darkfield masks: https://www.thingiverse.com/thing:4446434
- Pentax-adapter T2 to 22mm eyepiece tube: https://www.thingiverse.com/thing:3468342
- Plate holder (2-photon microscopy): https://www.thingiverse.com/thing:4697531
- Rotation insert 16×11 for inverted microscope: https://www.thingiverse.com/thing:4604394
- RPi-microscope for histology with focus-drive and x-y stage: https://www.thingiverse.com/thing:385308
- Scope magnifier throw levers: https://www.thingiverse.com/thing:5947013
- Side entrance microscope slide cuvette: https://3d.nih.gov/entries/3DPX-014335
- SPIM chamber: https://www.thingiverse.com/thing:4169370
- Spotlight for stereo microscope: https://www.thingiverse.com/thing:3000179
- Universal stage sample cover: https://www.thingiverse.com/thing:4207180
- USB microscope filter holder: https://www.thingiverse.com/thing:4624375
- Well plate microscope mount (for upright stands): https://www.thingiverse.com/thing:2910866
Microscope: Olympus-specific
- 50mm dust cap for the field lens of an Olympus BH2 microscope: https://www.thingiverse.com/thing:5419346
- 90mm petri dish holder for Olympus IX81 Prior stage: https://3d.nih.gov/entries/3DPX-012789
- A darkfield insert for the Olympus BH2 microscope: https://www.thingiverse.com/thing:5431323
- Adapter to mount Vanox darkfield condenser onto BH2 microscope: https://www.thingiverse.com/thing:5425778
- Bottom dust cap for binocular or trinocular viewing head of Olympus BH2 microscope: https://www.thingiverse.com/thing:5410351
- CH2-FH filter mount for CH2-DS darkfield central stop on Olympus CH-2 microscope: https://www.thingiverse.com/thing:3400092
- Darkfield insert for Olympus BH2 microscope: https://www.thingiverse.com/thing:5434202
- Darkfield insert for Olympus microscope: https://www.thingiverse.com/thing:5431252
- Dust cap for 23mm ocular tubes of Olympus BH2 microscope: https://www.thingiverse.com/thing:5413134
- Dust cap for the trinocular tube of Olympus BH2 microscope: https://www.thingiverse.com/thing:5411636
- Dust cap for viewing head recess of BH2 microscope: https://www.thingiverse.com/thing:5410353
- Microscope filter cube BX series: https://www.thingiverse.com/thing:2484257
- Microscope light control knob for Olympus CK30: https://www.thingiverse.com/thing:3080152
- Microscope sample/slide holder: https://www.thingiverse.com/thing:4754181
- Oblique insert for Olympus BH2 microscope: https://www.thingiverse.com/thing:5430689
- Oblique insert for Olympus BH2 microscope: https://www.thingiverse.com/thing:5430736
- Olympus adapter for ThorLabs MLS203-2 XY stage: https://www.thingiverse.com/thing:2255338
- Olympus BH-2 BHS slide holder: https://www.thingiverse.com/thing:4964747
- Olympus BHT/BHTU/BHS microscope stand cap (for BH and BH2): https://www.thingiverse.com/thing:3321971
- Olympus c-mount adapter: https://www.thingiverse.com/thing:4872851
- Olympus IX71 slide mount: https://3d.nih.gov/entries/3DPX-011946
- Olympus IX81 stage insert and lid for 2x35mm petri dishes: https://3d.nih.gov/entries/3DPX-012758
- Olympus IX81 stage insert and lid for IBIDI chamber slide: https://3d.nih.gov/entries/3DPX-012759
- Olympus VM stereo microscope parts: https://www.thingiverse.com/thing:4434812
- Petri dish holder (Olympus and other microscopes): https://www.thingiverse.com/thing:5437083
- Samsung Galaxy S8 microscope adapter for Olympus CH30: https://www.thingiverse.com/thing:3329665
- SM1 to 45mm optical filter adapter (Olympus IX71): https://www.thingiverse.com/thing:798174
- WHK eyepiece rack for Olympus BH2 microscope: https://www.thingiverse.com/thing:5406071
Microscopes: Leica-specific
- 1x microscope camera adapter c-mount for Leica DM microscopes: https://www.thingiverse.com/thing:2584651
- 25mm polarizer holder for microscope Nikon Epiphot: https://www.thingiverse.com/thing:4769898
- 75mm Glass slide and 35mm culture dish Leica microscope insert: https://3d.nih.gov/entries/3DPX-001726
- AO microscope adapter for 32mm filters: https://www.thingiverse.com/thing:4836163
- Culture plate stage adapter for Leica DMi8: https://www.thingiverse.com/thing:3329633
- Darkfield filter set: https://www.thingiverse.com/thing:633301
- Leica 1-inch filter deep hole w/3 clampscrews 2mm for DM6000: https://www.thingiverse.com/thing:189063
- Leica Cube FLIM Cal V4 hard PLA: https://www.thingiverse.com/thing:1123439
- Leica filter cube for DM IRBE microscope with dichroic flipped FLIM Calv4soft PLA: https://www.thingiverse.com/thing:139841
- Leica polariser insert: https://www.thingiverse.com/thing:3062120
- Leica SP8 filter cube – copy of SP8 fast FRAP 02: https://www.thingiverse.com/thing:1123461
- M25 Nikon/Leica objective case: https://www.thingiverse.com/thing:5395489
- Magnification knob Leica MZ 16 V2: https://www.thingiverse.com/thing:5559397
- Magnification knob Leica MZ16: https://www.thingiverse.com/thing:3915689
- Manual to automated stage conversion for Leica DMi8: https://www.thingiverse.com/thing:2778053
- Microscope filter cue: https://www.thingiverse.com/thing:3060644
- Microscope light holder for Leica stereomicroscope: https://www.thingiverse.com/thing:5329337
- Microscope plate adapters for Leica microscope: https://www.thingiverse.com/thing:5414677
- Microscopy analysis base to Leica confocal – ASTM_D638-14: https://www.thingiverse.com/thing:5262560
- Microscopy analysis base to Leica confocal – to filament and wire samples: https://www.thingiverse.com/thing:5338346
- Microtitre plate holder for Leica DMI 6000b microscope: https://3d.nih.gov/entries/3DPX-001727
- Nikon SMZ-U fine focus knob set
Microscopes: Zeiss-specific
- Carl Zeiss Jena Microsoft LifeCam adapter: https://www.thingiverse.com/thing:4230506
- Carl Zeiss Jena Technical stereo microscope rack: https://www.thingiverse.com/thing:5189531
- FEP-tube sample holder LS no 01 for Zeiss lightsheet: https://www.thingiverse.com/thing:4141197
- Filter holder for the Zeiss Lumar stereo fluorescent microscope: https://www.thingiverse.com/thing:2816047
- Multiwell-plate stage frame for Zeiss 47 17 24 stage (IM-35): https://www.thingiverse.com/thing:5421864
- Polarising filter holder for Zeiss inverted microscope: https://www.thingiverse.com/thing:2607475
- Prior H122 focus stepper driver support for Zeiss Axioscope A1: https://www.thingiverse.com/thing:33666
- Rotating slide adapter for zeiss confocal: https://3d.nih.gov/entries/3DPX-013011
- Tissue adaptor for Zeiss Z.1 LSFM: https://www.thingiverse.com/thing:3836158
- Zeiss 426126 microscope to Canon T mount adapter: https://www.thingiverse.com/thing:5670502
- Zeiss analyzer holder for axioskop: https://www.thingiverse.com/thing:5197647
- Zeiss axioskop microscope adapter for darkfield holder 1144 488: https://www.thingiverse.com/thing:5226488
- Zeiss axioskop microscope eyepiece cover: https://www.thingiverse.com/thing:5200069
- Zeiss Axiovert 200 insert to hold generic well plate: https://www.thingiverse.com/thing:628296
- Zeiss beamsplitter cube for microscopes: https://www.thingiverse.com/thing:2426035
- Zeiss -compatible Ph1, Ph2, and holder for 0.4 condenser: https://www.thingiverse.com/thing:4764868
- Zeiss CP.2 114mm lens cap: https://www.thingiverse.com/thing:3820327
- Zeiss lens cleaning wipe dispenser (needs spring): https://www.thingiverse.com/thing:4765397
- Zeiss lens wipe holder: https://www.thingiverse.com/thing:5236982
- Zeiss lightsheet sample holder standard L-shaped: https://www.thingiverse.com/thing:4171299
- Zeiss lightsheet sample holder standard L-shaped: https://www.thingiverse.com/thing:4171299
- Zeiss lightsheet sample holder stub medium: https://www.thingiverse.com/thing:4171547
- Zeiss LSM880 condenser cap: https://www.thingiverse.com/thing:5224412
- Zeiss polarizer and lambda plate 445226 to Zeiss axioskop 40 adapter: https://www.thingiverse.com/thing:5226513
- Zeiss polarizer and lambda plate 445226 to Zeiss Axioskop 40 adapter: https://www.thingiverse.com/thing:5226513
- Zeiss slide holder: https://www.thingiverse.com/thing:243580
- Zeiss-compatible frame for microwell plates: https://3d.nih.gov/entries/3DPX-016697
- Zeiss-compatible PH1, Ph2, and holder for 0.4 condenser: https://3d.nih.gov/entries/3DPX-016698
- Zeiss-compatible stage frame M: https://www.thingiverse.com/thing:4759996
Microscopes: Nikon-specific
- American Optical to Nikon condenser adapter: https://www.thingiverse.com/thing:3088633
- Fibre optic lamp holder for Nikon Optiphot 2: https://3d.nih.gov/entries/3DPX-011229
- Fine focus spur gear for Nikon model S microscope: https://www.thingiverse.com/thing:5005716
- F-mount/C-mount coupler/telescope/beam expander: https://3d.nih.gov/entries/3DPX-013073
- LabTek-II chamber adapter for Nikon slide holder: https://3d.nih.gov/entries/3DPX-015613
- M25 Nikon/Leica objective case: https://www.thingiverse.com/thing:5395489
- Microscope mount (Nikon) for a mini spectrophotometer: https://www.thingiverse.com/thing:33222
- Microscope Nikon TS2R or manual Ti2-A/U large bottle adapter: https://www.thingiverse.com/thing:5485332
- Nikon a 1.25 eyepiece scope: https://www.thingiverse.com/thing:4807501
- Nikon camera to Zeiss microscope adapter: https://www.thingiverse.com/thing:3017895
- Nikon condenser dark tube: https://www.thingiverse.com/thing:3088682
- Nikon DSLR camera to microscope ISO phototube adapter: https://www.thingiverse.com/thing:2985865
- Nikon DSLR microscope adapter: https://www.thingiverse.com/thing:4642087
- Nikon Eclipse microscope DIC holder: https://www.thingiverse.com/thing:5486300
- Nikon Eclipse Ti2 light shield: https://www.thingiverse.com/thing:3965959
- Nikon EPI analyzer: https://www.thingiverse.com/thing:5703136
- Nikon EPI rotatable analyzer: https://www.thingiverse.com/thing:5667953
- Nikon F mount microscope adapter: https://www.thingiverse.com/thing:5245146
- Nikon F-mount to 30mm microscope ocular mount: https://www.thingiverse.com/thing:1722898
- Nikon F-mount to Thorlabs 60mm cage plate: https://www.thingiverse.com/thing:4977738
- Nikon Labophot-2 fine focus gear v2: https://www.thingiverse.com/thing:5233980
- Nikon light ring: https://www.thingiverse.com/thing:2023953
- Nikon microscope filter holder – fits 36mm condenser: https://www.thingiverse.com/thing:4579175
- Nikon microscope filter slider: https://www.thingiverse.com/thing:3088682
- Nikon microscope humidity cover: https://www.thingiverse.com/thing:4733717
- Nikon microscope slide 75×25 stage mount: https://www.thingiverse.com/thing:2036022
- Nikon microscope slide 75×25 stage mount: https://www.thingiverse.com/thing:2036035
- Nikon SMZ-U camera port adapter – micro four thirds (M43): https://www.thingiverse.com/thing:4161613
- Nikon SMZ-U fine focus knob set: https://www.thingiverse.com/thing:4814210
- Nikon stackable rotatable polarizer for epi illuminator: https://www.thingiverse.com/thing:5633848
- Nikon stage insert: https://3d.nih.gov/entries/3DPX-000492
- Nikon TE2000 epi plug: https://3d.nih.gov/entries/3DPX-000435
- Nikon Ti2 eyepiece tube filter holder: https://3d.nih.gov/entries/3DPX-014904
- Nikon to Zeiss microscope adaptor: https://www.thingiverse.com/thing:3017895
- Nikon xxxPhot microscope slide holder: https://www.thingiverse.com/thing:4734545
- Parametric microfluidics device holder (Nikon stages): https://www.thingiverse.com/thing:827247
- Phase L condenser insert for Nikon Diaphot TMD: https://3d.nih.gov/entries/3DPX-012790
- Spacers between Nikon Ti2 and ASI stage: https://3d.nih.gov/entries/3DPX-014906