Reflections on Transcribing Multimodal Texts
26 March 2018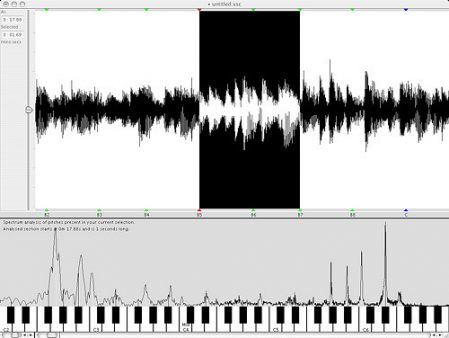
Since the election of Donald Trump in 2017 the media has often seemed to be in a continual state of shock at the brusque manner of the forty-fifth president’s speech. During the summer of 2017 I conducted a research project into transcription methods for multimodal discourse, contributing to the Changing Attitudes in Public Discourse study.
The aim of the project was to find an effective means of transcribing multimodal resources such as video recordings. An effective transcription method would support analysis into public discourse, especially how conversational behaviours like arrogance manifest in public discourse. Donald Trump is relevant as many people view him as a particularly arrogant communicator. Having a method to transcribe the communicative techniques of individuals like Trump will help identify and nullify elements of arrogance in public discourse.
The first step in selecting an appropriate transcription method was to identify which modalities – in this case communicative resources – would merit inclusion. Clearly, speech itself is one of the key modalities and has been the focus of most existing transcription methods. Where necessary, however, information showing specific pronunciation can be included. Verbal utterances that are not words such as laughter or coughing may also be important and were to be included. Similarly, gestures were set to be included as body language can be indicative of speaker attitudes. More subtle modalities such as eye gaze and facial expressions were also deemed potentially insightful for measuring participants’ attitudes. Eye gaze, for example, may suggest that a speaker is addressing one particular participant within a larger debate.
The modalities of speech, intonation, gesture, gaze and facial expression were considered to be requirements for the transcription method. It followed that the transcription method must be able to represent a range of modalities and information without becoming unclear. Furthermore, some of these modalities can be recorded through written description whereas some can be difficult to represent textually. For example, whilst we can write ‘participant A pointed towards participant B’, describing the nuances of a facial expression concisely may be challenging, or at worst misleading. It would be a benefit, therefore, for the chosen transcription method to be able to include still-frame images. The inclusion of images can help display modalities such as gesture, facial expression and even gaze without ambiguity. It also seemed important to consider that not all public debates being recorded for analysis would be alike. An effective transcription method, therefore, would be modular. A modular transcription method is flexible in its presentation, allowing the inclusion or exclusion of different modalities depending on whether they are appropriate.
Research into existing multimodal transcription methods revealed four existing styles of multimodal transcription. These are: playscript, tabular, timeline and image-based. Playscript transcriptions follow the same layout as their namesake – like reading a theatre play script, each speaker turn is listed chronologically down a page and each turn is usually numbered. This is the type of transcription that many will think of immediately if asked about speech transcription. Playscript transcriptions tend to focus extensively on speech, and other modalities such as gesture are often added in italic font between turns. It is very effective for analytic styles such as conversation analysis wherein speech itself is analysed in great detail, but it is difficult to represent a number of modalities simultaneously in a playscript transcription.
Tabular transcriptions separate each modality into a column or a row (depending whether the table is horizontally or vertically chronological), with simultaneous communicative events occupying the same level of the table. Tabular transcriptions are useful as they are very flexible due to their modularity. Columns or rows can be added or removed depending on how many modalities are required, and the separation of modalities allows each one to be considered in isolation if necessary. There may also be a column for images. Tabular transcription methods became one of the most suitable templates for multimodal transcription.
Timeline transcripts are laid out horizontally, with each modality occupying one horizontal axis. Heading the transcription are ongoing timestamps and usually there are images included periodically – every five seconds for example. Each modality is represented effectively in real time in relation to the timestamps, making it easy to see the duration of speech acts or gestures and which actions coincide. Regular images also lend gestures and expressions more clarity. Timeline transcriptions along with tabular transcriptions were exceptionally suitable.
The final transcription style, image-based, was uncommon and served a niche purpose. Images or even drawings of the event form the background, over which different modalities such as speech are superimposed. Directional arrows sometimes showed gaze. These were useful for showing specific situations, such as in instruction manuals, but it became immediately clear that the format would become unwieldy if a number of modalities were involved.
Both tabular and timeline transcription methods were appropriate for multimodal transcription. Both were modular, flexible and a number of modalities could be represented clearly. However, the timeline transcription was eventually deemed superior as the capacity to visually interpret the coincidence and duration of actions offered a significant advantage.
As the Changing Attitudes in Public Discourse project develops, timeline transcriptions will be a useful tool for transcribing multimodal public debates. Outlining a number of communicative modalities for direct analysis will aid identification of cues to arrogance and contribute to the creation of guidelines towards productive public debate.
Image from Ethan Hein on Flickr CC.BY 2.0
- July 2024
- June 2024
- May 2024
- April 2024
- March 2024
- February 2024
- January 2024
- December 2023
- November 2023
- October 2023
- September 2023
- August 2023
- July 2023
- June 2023
- May 2023
- April 2023
- March 2023
- February 2023
- January 2023
- December 2022
- November 2022
- October 2022
- September 2022
- August 2022
- July 2022
- June 2022
- May 2022
- April 2022
- March 2022
- February 2022
- January 2022
- December 2021
- November 2021
- October 2021
- September 2021
- August 2021
- July 2021
- June 2021
- May 2021
- April 2021
- March 2021
- February 2021
- January 2021
- December 2020
- November 2020
- October 2020
- September 2020
- August 2020
- July 2020
- June 2020
- May 2020
- April 2020
- March 2020
- February 2020
- January 2020
- December 2019
- November 2019
- October 2019
- September 2019
- August 2019
- July 2019
- June 2019
- May 2019
- April 2019
- March 2019
- February 2019
- January 2019
- December 2018
- November 2018
- October 2018
- September 2018
- August 2018
- July 2018
- June 2018
- May 2018
- April 2018
- March 2018
- February 2018
- January 2018
- December 2017
- November 2017
- October 2017
- September 2017
- August 2017
- July 2017
- June 2017
- May 2017