Deepfakes, Fake Barns, and Problems of Safe Belief
17 May 2021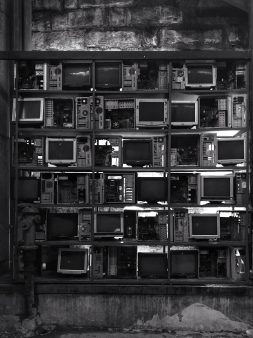
Every year, Queen Elizabeth II speaks to the UK in her annual Christmas Address. In her 2020 Address, the Queen not only spoke highly of NHS nurses, doctors, and other frontline workers during the Covid-19 pandemic, but she also expressed her desire to appear on the TV programme Strictly Come Dancing. Anyone who has watched a Christmas Address before would have quickly noticed that this confession was out of character for the Queen. In fact, her admission was not just out of character; it was entirely fake – Channel 4 had commissioned an ‘alternative’ Christmas Address that took the form of a so-called deepfake.
A portmanteau of ‘deep learning’ and ‘fake’, deepfakes are a relatively recent innovation but their presence is growing across the internet. Moreover, developers are using increasingly sophisticated technologies to create deepfakes to the extent that the acclaimed computer scientist and deepfake pioneer, Professor Hao Li, has recently warned that we’re ‘going to get to a point where there is no way that we can actually detect them [deepfakes] anymore’.[1] In an age of growing disinformation, ‘fake news’, and populism, deepfakes clearly add to the mounting problems we face when it comes to trusting online sources.
Several epistemologists have recently articulated a number of concerns about the role deepfakes could have on public discourse, undermining trust in videos, and reducing information-sharing.[2] However, I think deepfakes go one step further; deepfakes also pose a challenge to our very claims to gain knowledge from online videos. They do this by undermining what epistemologists call the Safety Principle. Roughly, this principle holds that a person knows something if their true belief could not too easily have turned out false, and it is often cashed out in terms of ‘modal’ or close possible worlds. To illustrate this, consider an (in)famous example first introduced by Alvin Goldman.
Barnaby is travelling by train through a particular part of the countryside, where several barns stand. During the journey, Barnaby spots one of these barns and admires its design. Unbeknownst to him, the barns in the field are mostly fake barn façades – the local council decided to erect numerous fake barns to attract tourists. As it turns out, though, the barn Barnaby is looking at is a real barn and not a fake. Does Barnaby know that he is looking at a real barn? Most epistemologists say that he does not.[3] One reason for this dissent is because his true belief that the barn is real could too easily have been false. Given how easily Barnaby could have been looking at a fake barn, it seems that in close possible worlds where Barnaby forms the same belief, it would turn out false. Hence, his belief is only true by luck. Since knowledge is taken to be incompatible with luck, Barnaby’s belief is unsafe and therefore fails to count as an instance of knowledge. Of course, fake barn cases are purely fictional but what if that were to change? To see how deepfakes put us in a similarly precarious position, let’s examine the technology behind them.
Fundamentally, deepfakes are a sophisticated form of facial manipulation akin to more familiar technologies such as attribute manipulation (also known as face retouching or editing) and expression swap (often referred to as face enactment). Often, deepfakes are grouped alongside forms of identity swap technology; indeed, this sort of technology is at work across popular face-swap apps and social media platforms such as Snapchat and Instagram, but also in in the special effects we watch in films and television. Nevertheless, what makes deepfakes stand out from these technologies is their reliance on powerful machine-learning technology, and the ‘deep’ in their name points towards the advanced ‘neural networks’ that generate the videos.
Most deepfakes are produced by so-called ‘Generative Adversarial Networks (GANs), which consist of two AI algorithms called the ‘generator’ and the ‘discriminator’. After being fed the same dataset of audio, video footage, and images, the adversarial component kicks in and the two algorithms ‘compete’ against each other – the generator creates new samples that are good enough to trick the discriminator, while the discriminator works to determine whether these new videos or samples are real. The result is an authentic-looking video capable of mimicking the voice, mannerisms, facial expressions, and speech inflections of one person before superimposing them onto another.
Although initially unsettling, one might argue that deepfakes seem distinct from fake barns because we can rely on our ears to pick up audio discrepancies or recognise unfamiliar voices coming from familiar faces. After all, the deepfake of Queen Elizabeth II was voiced by a professional actress. Unfortunately, this response is quite literally dwindling before our eyes. In 2017, Adobe and Princeton University created ‘VoCo’ technology, which allows video creators to alter the content of an audio recording by typing words into a transcript. By analysing voice samples of a target speaker, VoCo algorithms are able to synthesise what the speaker’s voice would sound like were they to say the things written into the transcript. In the hands of deepfake purveyors, this creates two more problems for us.
First, VoCo technology now means that deepfakes no longer need to rely on actors to provide compelling voices. If a deepfake generates the exact voice of the target speaker, our initial response is to take this at face value as we might with regularly testimony and accept what we hear. So long as there no defeating reasons to dismiss the testimony, VoCo technology brings our ears closer to redundancy in the search for deepfakes. Second, creators can now dispense with audio clips that might have initially given a deepfake away because they no longer need to correctly map different mouth movements onto the target. In turn, this removes any ability we might have previously had in picking up facial discrepancies in deepfakes.
Where does this leave us? A worrying conclusion we can draw from this is that deepfakes will eventually become indistinguishable from other online videos we watch. When or if this happens, our ability to discern true videos from false ones will become much harder, much like Barnaby’s ability to tell real barns from fake barns. There’s an important upshot to this to this. In addition to accumulating more false beliefs from videos than we currently do, sophisticated deepfakes could also place us in a situation like Barnaby’s: just as the fake barn façades jeopardise the safety of his true belief, the spread of deepfakes means that, despite forming a true belief about an event or person via an online video, all too easily could we have formed the same belief across close possible worlds, and it be a deepfake. Put simply, deepfakes are increasingly rendering our true beliefs from videos unsafe, and this leaves us with the prospect that we might soon lose any claims to knowledge about what we watch online.
So, the next time you’re surfing the internet and stumble across a video you take to be true, think: do I know what I’m watching is true?
[1] K. Stankiewicz, ‘‘Perfectly Real’ Deepfakes Will Arrive In 6 Months to a Year, Technology Pioneer Hao Li Says’, CNBC, 2019.
[2] See, for example, R. Rini ‘Deepfakes and the Epistemic Backdrop’, Philosophers’ Imprint, 20:24, 2020, and D. Fallis ‘The Epistemic Threat of Deepfakes’, Philosophy and Technology, 2020.
[3] Despite what epistemologists say, a number of studies show that non-specialists are more inclined to grant people like Barnaby with knowledge about the barn. See, for example, D. Colaço et al. ‘Epistemic Intuitions In Fake-Barn Thought Experiments’, Episteme, 11:2, 2014. For the original fake barn case, see A. Goldman, ‘Discrimination and perceptual knowledge’, Journal of Philosophy, 73, 1976, pp. 771-791.
- July 2024
- June 2024
- May 2024
- April 2024
- March 2024
- February 2024
- January 2024
- December 2023
- November 2023
- October 2023
- September 2023
- August 2023
- July 2023
- June 2023
- May 2023
- April 2023
- March 2023
- February 2023
- January 2023
- December 2022
- November 2022
- October 2022
- September 2022
- August 2022
- July 2022
- June 2022
- May 2022
- April 2022
- March 2022
- February 2022
- January 2022
- December 2021
- November 2021
- October 2021
- September 2021
- August 2021
- July 2021
- June 2021
- May 2021
- April 2021
- March 2021
- February 2021
- January 2021
- December 2020
- November 2020
- October 2020
- September 2020
- August 2020
- July 2020
- June 2020
- May 2020
- April 2020
- March 2020
- February 2020
- January 2020
- December 2019
- November 2019
- October 2019
- September 2019
- August 2019
- July 2019
- June 2019
- May 2019
- April 2019
- March 2019
- February 2019
- January 2019
- December 2018
- November 2018
- October 2018
- September 2018
- August 2018
- July 2018
- June 2018
- May 2018
- April 2018
- March 2018
- February 2018
- January 2018
- December 2017
- November 2017
- October 2017
- September 2017
- August 2017
- July 2017
- June 2017
- May 2017