AI in healthcare: the outlook in Wales
31 October 2024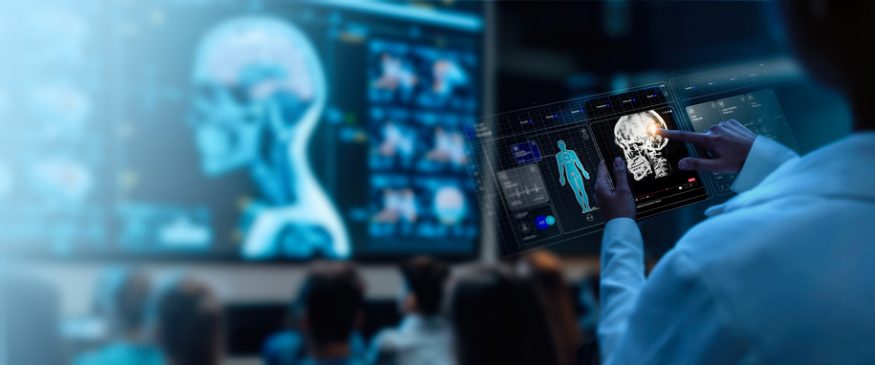
In the last year or so, we’ve heard many dire warnings about AI taking jobs, even taking over the world and plotting our very extinction. But where is the reality in all the hype?
The speed at which large language models, or LLMs, have proliferated and become standard tools used by a range of people, means we can’t afford to ignore this transformative technology. We also cannot ignore a health service and social care system that is at breaking point. The real question is, how can we use this technology to improve care, and does it have the potential to do more than that? What more would we like it to do? Could we reimagine health and social care in a data-rich and tech-savvy future?
AI in Action
In Buckinghamshire, AI-linked sensors on kettles and fridges identify changes in eating and drinking habits of vulnerable patients, flagging them to a non-clinical Onward Care team who contact the patients, and can solve 95% of issues without clinical escalation.
In parts of Birmingham, an Early Intervention trial took place that avoided 20,000 overnight hospitalizations, saved 120,000 “bed days” per year and created £26.7M of financial benefit by using AI to predict the 5% of the population most at risk of admission. Staff were able to intervene with these patients prior to admission, offering social care assessments, medication reviews, and social prescribing. This integrated approach is now “business as usual”.
In these scenarios, the AI is a tool that frees up people to focus on where they can best add value, rather than removing people from the system entirely. Importantly, these interventions also don’t rely on the public themselves using specific apps or owning smartphones, which we know excludes some of the most vulnerable in our communities. Instead, the focus is on data-led, integrated care that makes the most of existing resources. Another key aspect is that the AI systems are advisory only – they are prompting the service providers to action, suggesting interventions, but not dictating them. AI is not infallible, and so there must always be human decision making involved in the process.
Predictive AI
Predictive AI, which can be considered to include machine learning, is fundamentally a means of synthesising volumes of data that are impossible for humans to process. Between Dec 2022 and Nov 2023, the NHS in England performed over 45 million imaging tests, including ultrasounds, CT scans, and MRIs. Researchers at Cardiff University are already working with Radiologists to use predictive AI to sort through mammograms to highlight only unusual scans for clinical attention, using AI to directly reduce clinical load.
It is these types of task that AI has the immediate ability to support – automation, data analysis, mapping, and prediction. AI can find new genetic associations for diseases, and new avenues for treatment. Natural language processing allows narrative information in clinical notes to be used as a rich data source, and there is a role for generative AI in tasks as fundamental (but also time consuming) as automating notes and history-taking. AI could suggest treatment options, look at a patient’s entire clinical history in moments, link multimodal data such as imaging and genetic sequencing, offering clinical staff holistic summaries, and saving patients from having to repeatedly tell their stories.
According to a recent report from the Health Foundation there is support from both the public and NHS staff for AI to be used in these sorts of ways. Indeed, AI is already being used in NHS 111 to help automate patient triaging using a medically-trained probabilistic network with a conversational AI chat bot at the front-end, preparing a complete case review for clinicians to use for call backs.
However, AI-powered interventions might also result in increased care needs. Cardiff researchers recently published a preprint that explores using AI to predict the development of mental health problems. If this were implemented, could existing services cope with an increase in demand of even just one or two percentage points?
Regulation
A considerable issue both in the use of AI and the acceptance of its use by the public is accountability and transparency in decision making. While “black box” AI, including ChatGPT, is powerful, AI in public services must be responsible, transparent, and accountable, even at the expense of performance. To this end, last month the Welsh Government endorsed the Algorithmic Transparency Recording Standard (ATRS), which was developed by the Centre for Digital Public Services and aims to record and share what algorithmic tools are being used by public sector organisations for decision making.
However, AI, whether generative or predictive, is only as good as the data it is trained on. “Rubbish in; rubbish out” is the data scientist’s mantra. When the data in question is people’s medical information, their conditions, their lives, the responsibility for managing this data cannot be understated. How do we ensure cyber security, and cyber resilience? Data standards, data governance, data ethics. These topics aren’t as exciting as generative AI, but they are the bedrock upon which a digital health and social care service must be built. There is opportunity here to learn from other industries – such as logistics and manufacturing, which are highly digitised, to share best practices.
Data sharing
The pandemic saw data sharing in unprecedented ways, including the instant sharing of diagnostic information with patients without the intervention of a health care practitioner. When we empower patients with information, what is our responsibility to educate them? What information can be delivered straight to phones, and what can’t? Who decides, and is it the same decision across the whole nation? Is it more ethical to make a patient wait longer to receive information, when the delay itself is distressing?
The fundamental principle of AI is that it learns and improves as more data is fed into it, and so our use of AI must undergo the same constant learning and improvement. We cannot implement these systems and then ignore them, we must monitor, assess, reflect, and change our approaches as necessary. Data-driven systems lend themselves to monitoring and reporting; the important task for us is to pay attention.
AI has been with us for over 40 years, but its capabilities didn’t grow in a linear fashion. The models got larger without any real improvements in accuracy for a long time, until, finally, a data threshold was reached, and accuracy skyrocketed. Data driven systems are at their most powerful when they have the largest quantities of data. Integrated, unified, cohesive platforms rolled out across the entire nation are more powerful than numerous isolated pilots, however innovative. If we can learn from others, and share innovative approaches, we’ll have the strongest chance of investing the resources available to us to deliver the best outcomes for our population.
Author: Angharad Watson, Digital Transformation Innovation Institute Manager