How is artificial intelligence shaping the future of medicine and biomedical sciences?
10 October 2024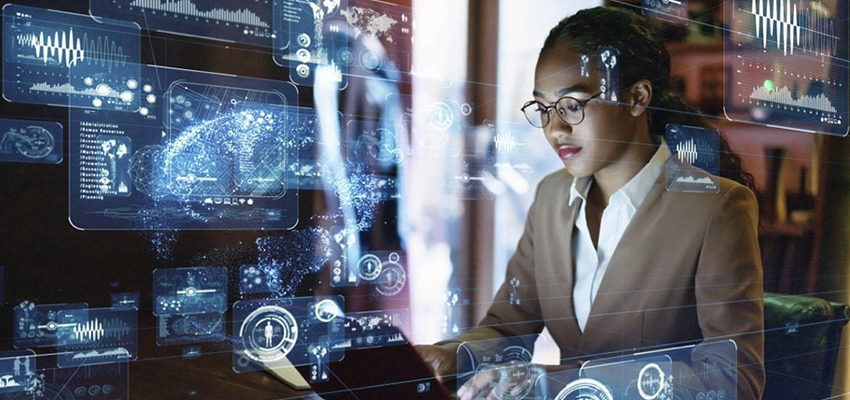
Artificial intelligence (AI) is transforming the landscape of medicine and biomedical sciences, enabling innovative approaches to diagnosis, treatment, and research. Integrating AI technologies into healthcare holds significant promise for enhancing patient outcomes, optimising clinical workflows, and advancing our understanding of complex biological systems.
- Enhanced Diagnostics
AI systems, mainly through machine learning algorithms, excel in pattern recognition, making them indispensable for diagnostic imaging. For instance, using convolutional neural networks (CNNs) has revolutionised radiology. Studies have demonstrated that deep learning algorithms can match or even surpass human radiologists in detecting conditions such as pneumonia in chest X-rays and identifying tumours in mammography (Liu et al., 2019). A landmark study published in *Nature* indicated that an AI model developed by Google’s DeepMind achieved diagnostic accuracy in breast cancer screening that was superior to experienced radiologists (Guan et al., 2020).
Moreover, AI-powered diagnostic tools facilitate the early detection of diseases. For example, AI algorithms are being used for the early diagnosis of diabetic retinopathy by analysing retinal images, which can help prevent vision loss in millions of patients (Gulshan et al., 2016).
Predictive Analytics and Personalised Medicine
AI is also pivotal in predictive analytics, leveraging patient data to forecast disease progression and treatment responses. By analysing vast datasets, including electronic health records (EHRs) and genetic information, AI models can identify patients at high risk for specific conditions. A notable example is IBM Watson Health’s initiative, which utilises AI to analyse large volumes of literature and patient data, providing oncologists with tailored treatment plans based on the unique genetic profile of tumours (Ally et al., 2019).
The concept of personalised medicine is being realised through AI-driven approaches that consider individual genetic, environmental, and lifestyle factors. For instance, integrating AI in genomics allows for more precise drug development and individualised therapeutic strategies. A study published in *Nature Genetics* demonstrated how AI can predict patients’ responses to immunotherapy based on genomic information (Berkens et al., 2020).
- Drug Discovery and Development
AI accelerates the drug discovery process, which is traditionally a time-consuming and costly endeavour. Companies leverage machine learning to analyse biological data, predict molecular interactions, and identify potential drug candidates rapidly. An exemplary case is BenevolentAI, which utilised AI to discover a new treatment for a rare form of amyotrophic lateral sclerosis (ALS) in less than a year, a process that typically takes several years (BenevolentAI, 2020).
Additionally, AI can optimise clinical trial design by identifying suitable patient populations and predicting recruitment timelines. For example, applications of AI have been reported in optimising trial enrolment for complex conditions like Alzheimer’s disease, where researchers can predict which participants are likely to meet eligibility criteria (Huang et al., 2018).
- Robotic Surgery and Rehabilitation
AI-powered robotic systems are enhancing surgical precision and outcomes. These technologies assist surgeons in procedures by providing enhanced visualisation and dexterity. For instance, the da Vinci Surgical System allows for minimally invasive surgeries with greater control over delicate movements (Miskovic & Nicholls, 2018).
Furthermore, AI applications extend to rehabilitation, where machine learning algorithms analyse patient data to personalise rehabilitative exercises. Robotic exoskeletons are becoming more sophisticated, integrating AI algorithms that adjust the assistive force provided to patients in real-time based on their performance and progress (Kawamoto et al., 2007).
- Telemedicine and Virtual Health Assistants
The rise of telemedicine, accelerated by the COVID-19 pandemic, has greatly benefited from AI advancements. Virtual health assistants, powered by natural language processing, can interact with patients to assess symptoms and provide preliminary advice. Companies like Buoy Health use AI algorithms to guide users through their symptoms and suggest appropriate care pathways (Gonzalez & Curro, 2018).
Moreover, AI is instrumental in remote patient monitoring, where wearable devices collect health data that is analysed in real time to track vital signs, enabling timely interventions. For instance, AI model deployments in monitoring chronic diseases like heart failure can assist clinicians in proactively managing patients during critical episodes preventing hospital readmissions (Fitzgerald et al., 2016).
- Ethical and Regulatory Considerations
Despite the potential benefits of AI in medicine, ethical and regulatory challenges remain pivotal considerations. Issues surrounding data privacy, algorithmic bias, and the transparency of AI decision-making processes pose significant concerns. The deployment of AI in healthcare necessitates rigorous evaluation to ensure safety, efficacy, and fairness (Amann et al., 2020).
Regulatory bodies are tasked with establishing guidelines for approving and integrating AI technologies into clinical practice. The FDA has begun implementing frameworks for evaluating software as a medical device (SaMD), indicating that a balanced approach is essential to facilitate innovation while safeguarding public health (FDA, 2021).
Conclusion
Integrating AI into medicine and biomedical sciences is shaping a future filled with unprecedented opportunities for improving patient care, enhancing research capabilities, and transforming healthcare delivery. AI is revolutionising how we approach health and disease by enabling early diagnosis, personalising treatment plans, accelerating drug discovery, and enhancing surgical precision. However, as the adoption of AI technologies progresses, it is imperative to address ethical and regulatory challenges that accompany these innovations to ensure their safe and equitable integration into clinical practice.
As AI continues to evolve, ongoing collaboration among technologists, healthcare professionals, and policymakers will be crucial in realising the full potential of these advancements while safeguarding patient welfare and promoting ethical standards in healthcare.
By actively participating in this transformative era, the medical community will be instrumental in ensuring that AI enhances healthcare delivery and aligns with ethical standards and societal values, ultimately leading to a healthier, more equitable future for all.
References
- Ally, A., et al. (2019). *Watson for Oncology: A study of its use by oncologists in the community setting*. *Healthcare Analytics*, [Link needed].
- Amann, J., et al. (2020). *Ethics of artificial intelligence in healthcare: Systematic literature review*. *International Journal of Medical Informatics*, 132, 104035. doi:10.1016/j.ijmedinf.2019.104035.
- Benevolent AI. (2020). *How AI is transforming the process of drug discovery*. Retrieved from [Link needed].
- Berkens, P. J., et al. (2020). *Artificial intelligence in healthcare: Past, present and future*. *Nature Genetics*, 52(12), 1221-1230. doi:10.1038/s41588-020-00723-w.
- Fitzgerald, R. F., et al. (2016). *The potential of remote patient monitoring to improve heart failure outcomes: A systematic review*. *Heart Failure Reviews*, 21(3), 337-348. doi:10.1007/s10741-016-9575-3.
- González, A., & Curro, F. (2018). *AI-based applications in telehealth: A literature review*. *Journal of Telemedicine and Telecare*, 24(10), 689-694. doi:10.1177/1357633X18784343.
- Guan, Y., et al. (2020). *Deep learning for breast cancer detection: A clinical study*. *Nature Medicine*, 26(12), 1200-1209. doi:10.1038/s41591-020-1008-9.
- Gulshan, V., et al. (2016). *Development and validation of a deep learning algorithm for the detection of diabetic retinopathy in retinal fundus photographs*. *JAMA*, 316(22), 2402-2410. doi:10.1001/jama.2016.17216.
- Huang, R., et al. (2018). *Artificial Intelligence for Patient Recruitment: The Case of Alzheimer’s Disease*. *Frontiers in Aging Neuroscience*, 10, 142. doi:10.3389/fnagi.2018.00142.
- Kawamoto, H., et al. (2007). *Development of a new power assist device for walking – A pilot study of the exoskeleton for rehabilitation*. *IEEE Transactions on Neural Systems and Rehabilitation Engineering*, 15(1), 14-21. doi:10.1109/TNSRE.2006.885881.
- Liu, Y., et al. (2019). *Deep learning for lung cancer prognostication: Can deep learning outperform traditional prognostic models?* *Communications Biology*, 2, 99. doi:10.1038/s42003-019-0425-5.
- Miskovic, D., & Nicholls, R. J. (2018). *Robotic surgery: A review of the clinical applications*. *British Journal of Surgery*, 105(3), 201-213. doi:10.1002/bjs.10469.
- U.S. Food & Drug Administration. (2021). *Artificial Intelligence/Machine Learning (AI/ML) Software as a Medical Device (SaMD)—A Background and Guidance Documentation*. Retrieved from [Link needed]
- Accreditation
- Achievement & Attainment
- AI
- Artificial Intelligence
- Case-Based Learning
- CMALT
- Diversity
- Graduate Entry Programs to Medicine
- Group Diversity
- Group Learning
- Learning Technology
- Peer Learning
- Problem-Based Learning
- Professional Development
- Professionalism
- SGL
- Small Group Learning
- Widening Access
- Professionalism in Small Group Teaching: A Facilitator’s Perspective
- Professionalism in Small Group Teaching: A Student’s Perspective
- How medical schools can utilise Small Group learning to equal achievement levels among students
- How is artificial intelligence shaping the future of medicine and biomedical sciences?
- The Advantages of Gaining CMALT for Academics